Understanding YouTube Algorithms: Increase Your Views & Engagement
Land Your First Data Science Job
A proven roadmap to prepare for $75K+ entry-level data roles. Perfect for Data Scientist ready to level up their career.
TL;DR
- YouTube's algorithm uses advanced machine learning to personalize content recommendations, significantly impacting user engagement and content discovery.
- Understanding engagement metrics like watch time and interaction is crucial for creators to optimize video visibility and grow their channels effectively.
- Ethical considerations like data privacy, moderation of misinformation, and algorithmic bias pose ongoing challenges that YouTube continues to address.
- Future developments in AI and transparency efforts aim to balance user satisfaction with responsible content promotion.
Introduction
What is YouTube's Algorithm?
YouTube's algorithm refers to the proprietary system used by YouTube to determine which videos to recommend to viewers. The primary purpose of this algorithm is to deliver personalized and engaging content, thereby increasing viewer satisfaction, watch time, and retention. For viewers, the algorithm enhances user experience by curating videos tailored to individual interests and viewing history. For creators, understanding the algorithm is crucial as it influences video visibility, reach, and channel growth. Advertisers also benefit since the algorithm ensures their advertisements are targeted effectively, reaching the most relevant audiences.
Brief History and Evolution
Initially, YouTube's ranking systems were relatively straightforward, primarily based on simple metrics such as clicks and view counts. In 2012, a significant shift occurred when YouTube started prioritizing watch time, emphasizing viewer engagement and retention rather than merely video clicks. Another pivotal milestone was in 2016 when YouTube began heavily integrating advanced machine learning and artificial intelligence to further personalize recommendations and predictions of user interest. More recently, in 2020, YouTube evolved its algorithm further by increasingly incorporating direct user feedback—such as survey responses and explicit user signals—to refine recommendations and enhance viewer satisfaction.
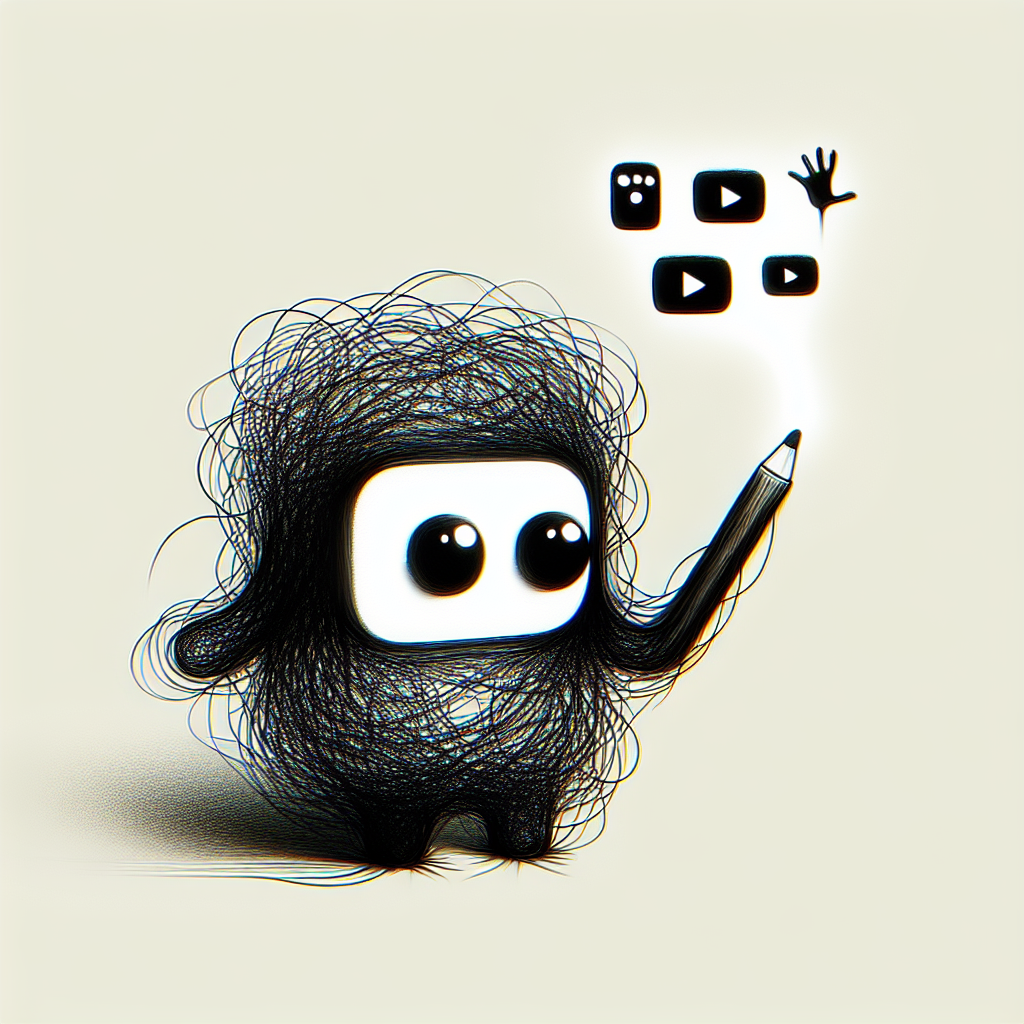
Technical Foundations of YouTube's Algorithm
Algorithmic Models and AI
YouTube's recommendation system relies heavily on advanced machine learning frameworks and deep learning techniques. These frameworks analyze vast amounts of user-generated data, enabling the platform to predict user preferences and behavior patterns. Deep neural networks, specifically recurrent neural networks (RNNs) and convolutional neural networks (CNNs), are commonly deployed to process complex data, including video content features, user interactions, and viewing history.
Personalization is achieved through data analytics, where individual user interactions—such as likes, dislikes, watch durations, and search queries—are collected and analyzed. This granular approach allows YouTube to curate highly personalized content feeds, delivering content tailored explicitly to the user's demonstrated interests and viewing habits.
Data Collection and Privacy Concerns
YouTube's algorithm extensively collects user data, encompassing viewing history, user interactions, search terms, geographic locations, device types, and engagement patterns. This comprehensive data collection helps refine recommendations but raises significant ethical implications and privacy concerns. Users often have limited awareness of the scope and depth of data collected, leading to growing scrutiny and debate surrounding algorithm transparency.
The ethical implications of YouTube's data collection practices are increasingly being discussed, particularly regarding user consent, data security, and potential algorithmic biases. Wired has highlighted concerns related explicitly to data privacy and the opacity of YouTube's recommendation algorithms, pointing out that the lack of transparency can negatively impact creators, users, and broader societal trust (Wired).
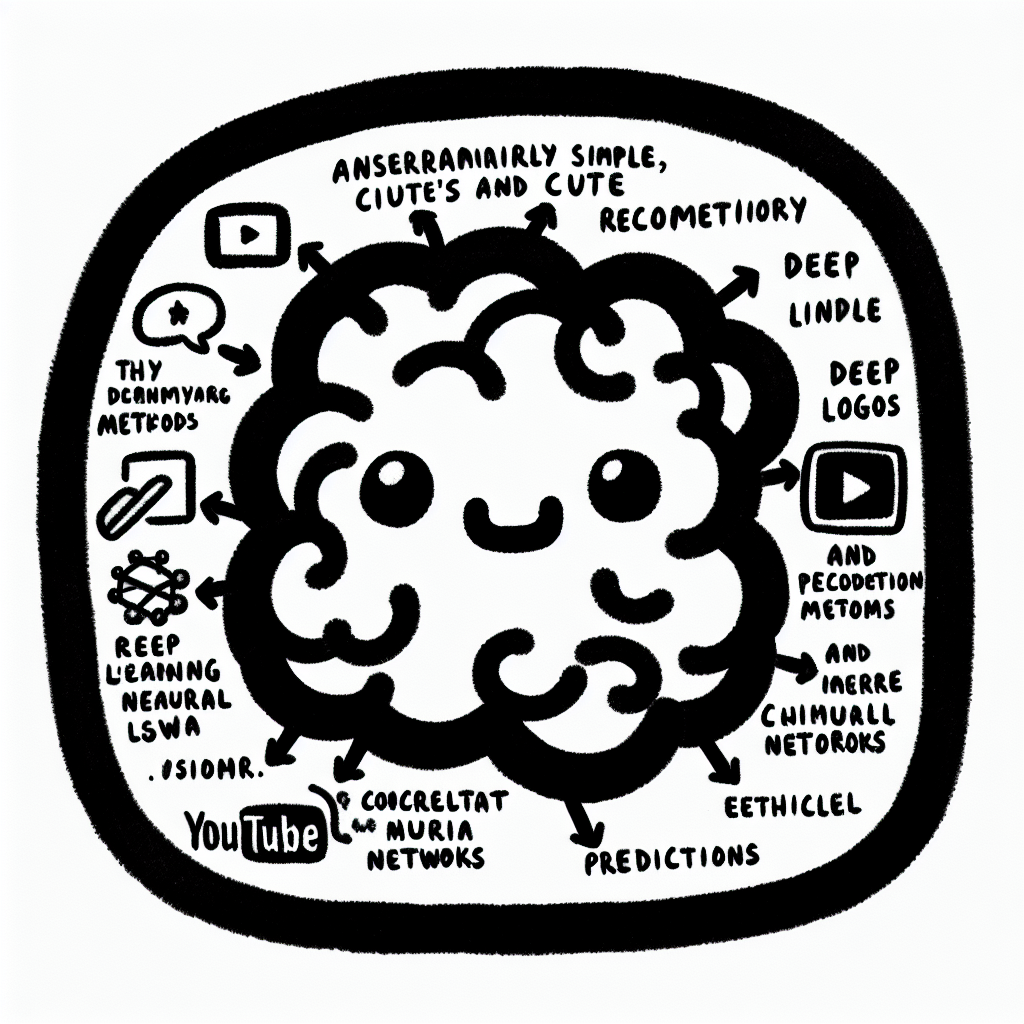
Core Components Influencing the YouTube Algorithm
Viewer Retention
Viewer retention, specifically watch time, is a critical factor in the YouTube algorithm. YouTube prioritizes content that keeps viewers engaged for longer durations, as increased viewing time indicates content value and relevance. Several factors influence viewer retention, including video length, production quality, and pacing. Videos that effectively balance duration with audience interest and maintain consistent pacing tend to retain viewers better, signaling to the algorithm that the content is worthy of broader promotion.
Engagement Metrics
Engagement metrics such as likes, dislikes, comments, shares, and click-through rates play a significant role in content ranking on YouTube. When viewers interact actively with videos—whether positively or negatively—it indicates strong viewer interest and relevance, prompting the algorithm to promote the content further. Content receiving higher levels of engagement is more likely to appear in recommendations, search results, and suggested video lists, enhancing visibility and reach.
Metadata Optimization
Metadata elements—including titles, thumbnails, descriptions, and tags—are crucial for optimizing video discoverability and performance within the YouTube algorithm. Titles and thumbnails serve as first impressions, significantly influencing click-through rates. Accurate and compelling descriptions and relevant tags help YouTube understand video content context, improving indexing accuracy. Best practices for effective metadata include using clear, concise titles, visually appealing thumbnails that accurately represent content, detailed descriptions with targeted keywords, and carefully chosen, relevant tags. Properly optimized metadata increases the likelihood of content discovery and promotion by the YouTube algorithm.
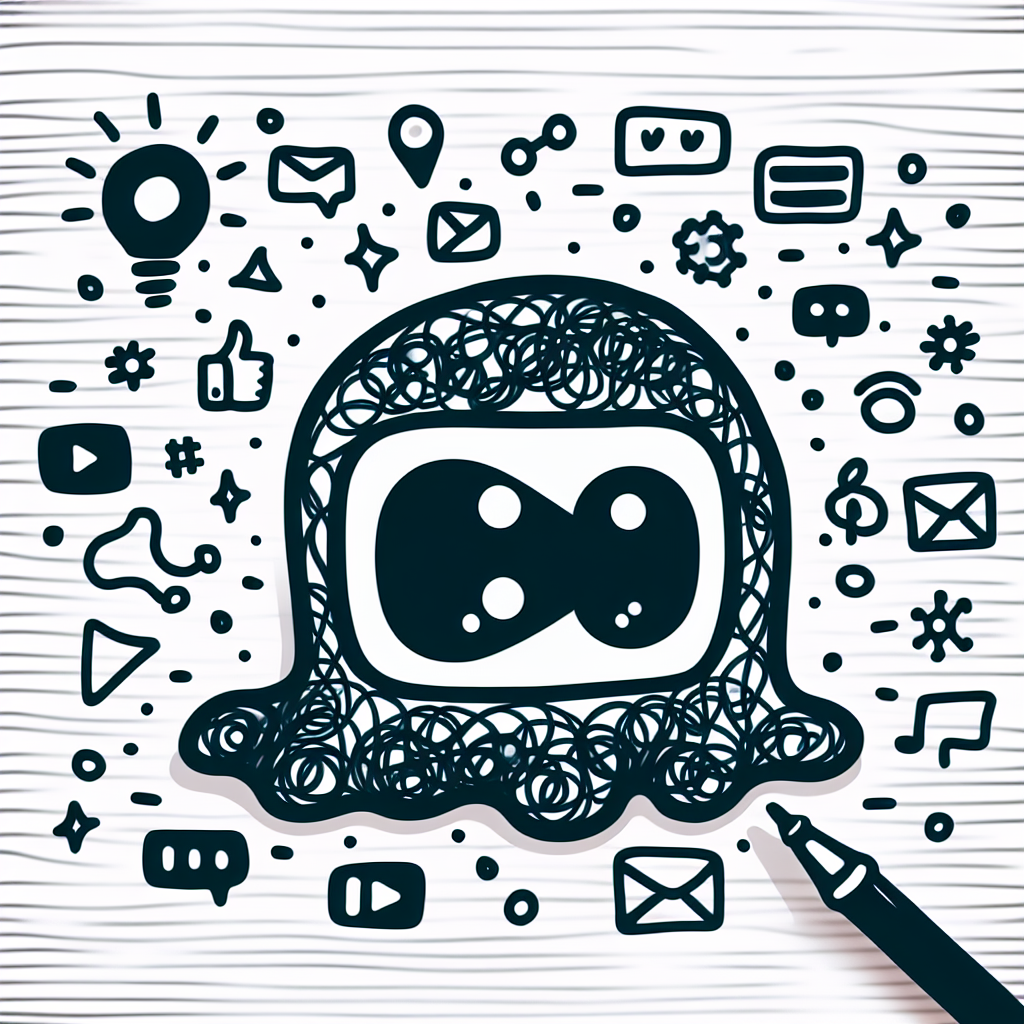
How the Algorithm Shapes Viewer Behavior
Content Discovery and Recommendations
YouTube's algorithm significantly shapes what viewers choose to watch through its personalized recommendation system. Recommended videos, appearing prominently alongside or below the currently viewed content, heavily influence viewers' subsequent choices, guiding their exploration and consumption patterns. These recommendations are generated based on the user's viewing history, engagement signals (such as likes, comments, and shares), and overall viewing time. Through this mechanism, the platform effectively directs attention toward specific content, creating distinct viewing patterns across its vast user base.
Trending topics and search results also play a pivotal role in shaping viewer behavior. By promoting videos that are currently popular or widely discussed, YouTube propels certain content into the spotlight, attracting broader audiences and influencing viewer interests. According to an analysis by The New York Times, engagement-driven recommendations can sometimes lead viewers toward sensational or misleading content, highlighting the algorithm's potential to unintentionally amplify misinformation.
Psychological Impact on Viewers
The constant exposure to algorithmically-curated content influences viewers' psychological tendencies, impacting their viewing habits and consumption preferences. Users frequently find themselves consuming videos aligned with their established interests, reinforcing existing beliefs and opinions. This phenomenon, known as an algorithm-driven echo chamber, can limit the viewer's exposure to diverse perspectives, reinforcing confirmation biases and potentially narrowing their worldview.
Moreover, the algorithm's personalized approach creates a feedback loop, where past viewing decisions shape future recommendations, further reinforcing specific viewing patterns. As a result, viewers may experience limited content diversity, potentially affecting their understanding of complex issues and their openness to alternative viewpoints.
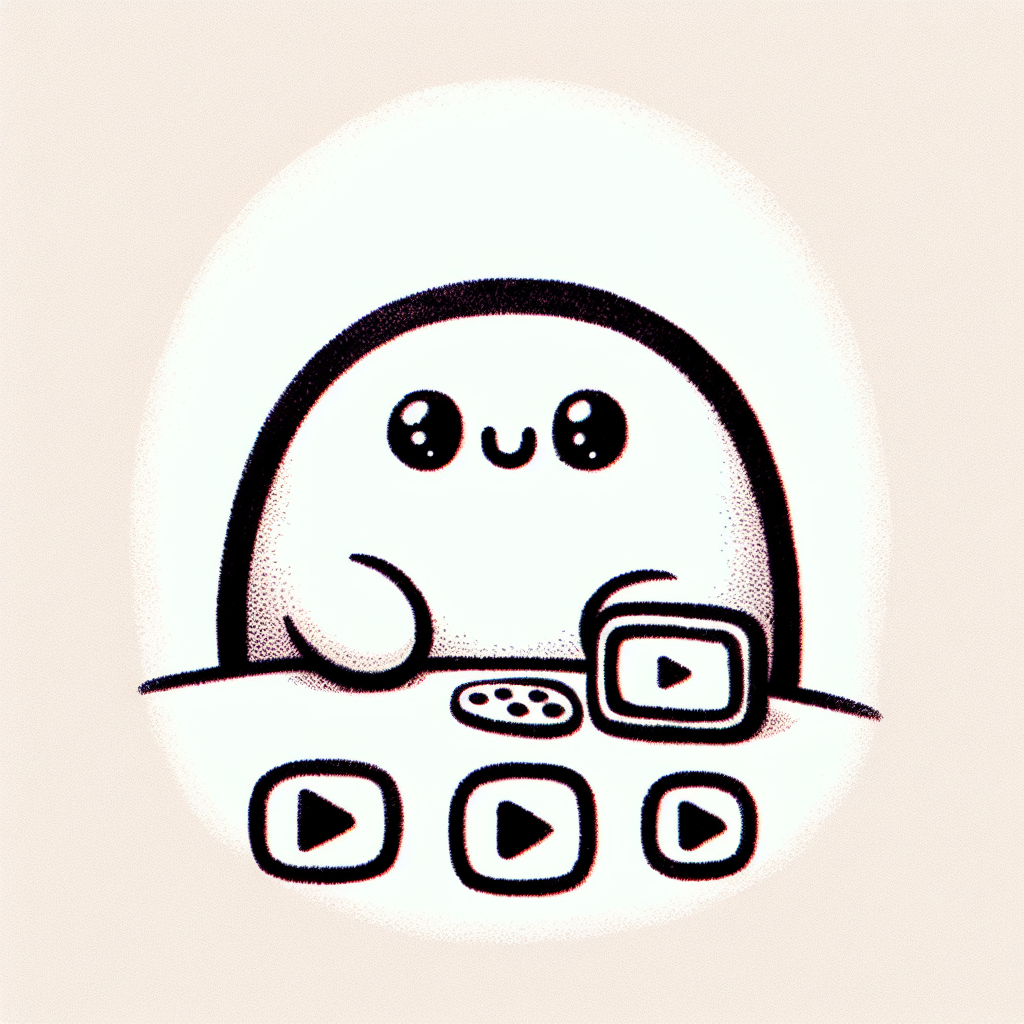
Algorithm's Impact on Content Creators
Visibility and Monetization
YouTube's algorithm has a significant effect on content creators, especially concerning video visibility and earnings. Videos ranked highly by the algorithm benefit from increased visibility, appearing more frequently in user recommendations and search results. Conversely, videos that fail to align with algorithmic preferences can become nearly invisible, severely impacting a creator's audience reach and monetization potential. Monetization strategies must therefore align closely with algorithmic trends. Creators increasingly tailor their content to fit algorithm-driven recommendations, aiming to boost views, engagement, and consequent ad revenue. According to Wired, YouTube's algorithm directly influences both the visibility and earnings of creators, underscoring the importance of algorithmic alignment in content strategy (Wired discusses the direct impact of YouTube's algorithm on creators' visibility and earnings).
Adapting Content Strategies
Adapting to the algorithm requires creators to actively identify and leverage emerging trends. By remaining aware of trending topics and user interests, creators can produce timely, relevant content that aligns with algorithmic priorities. Maintaining consistency and frequency of content production is also essential; regular posting signals to the platform's algorithm that a channel is active and engaging, increasing the likelihood of content promotion. Additionally, analytics tools provided by YouTube offer creators valuable insights into viewer behavior, allowing them to assess performance data and adjust strategies accordingly. Understanding these metrics helps creators make informed decisions about future content direction and timing.
Case Studies of Successful Adaptation
Several creators have successfully navigated algorithmic changes by strategically adapting their content production methods. For instance, prominent YouTubers have found success by swiftly responding to trending topics, effectively aligning their content with algorithm preferences and maximizing their visibility. Creators like MrBeast and Marques Brownlee (MKBHD) consistently demonstrate the effectiveness of aligning content strategy with algorithmic cues—such as sustained viewer engagement, watch-time optimization, and regular upload schedules. These examples provide valuable lessons for aspiring creators, highlighting the importance of flexibility, responsiveness, and a data-driven approach in navigating YouTube's ever-evolving algorithm.
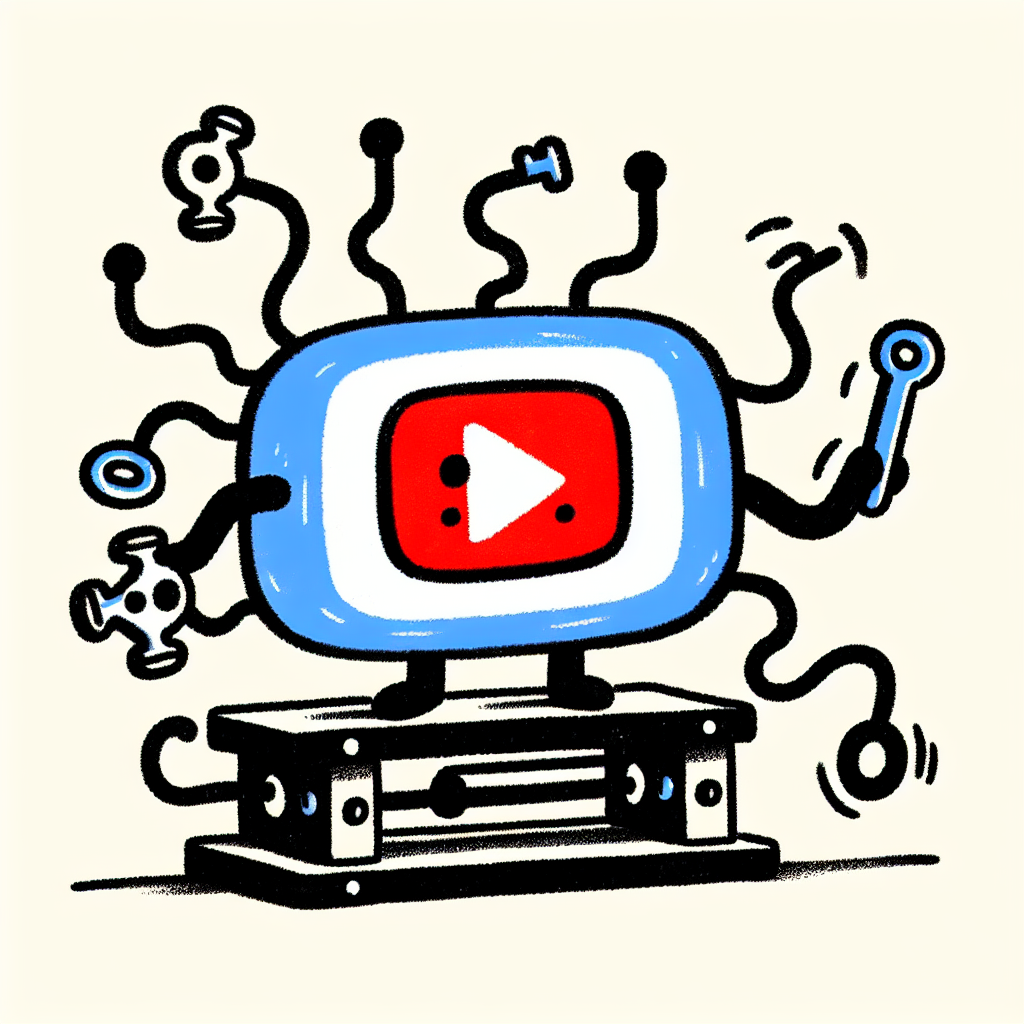
Ethical and Social Implications of YouTube's Algorithm
Content Moderation and Misinformation
YouTube's algorithm plays a significant role in moderating and promoting content, directly influencing what users see and interact with on the platform. While the algorithm aims to enhance user engagement by recommending relevant content, it has also been scrutinized for its potential to amplify misinformation. YouTube has implemented several algorithmic changes intended to reduce the spread of misleading or harmful content, shifting its focus towards authoritative sources and verified information. However, balancing content moderation with freedom of expression remains challenging. According to The Verge, algorithmic adjustments have had mixed results, with some improvements in misinformation reduction but ongoing concerns about transparency and effectiveness.
Algorithmic Bias and Diversity
Another significant ethical concern related to YouTube's algorithm is the presence of algorithmic bias, which can affect fairness and representation on the platform. Biases inherent in recommendation algorithms may unintentionally disadvantage marginalized creators, limiting the visibility and reach of diverse content. This has implications not only for creators' success but also for audience exposure to varied perspectives and narratives. Critics argue that algorithms can reinforce existing societal biases by disproportionately recommending content from dominant groups or mainstream viewpoints. Addressing algorithmic fairness is vital to fostering an equitable environment that empowers a diverse range of creators and ensures a richer, more inclusive content landscape.
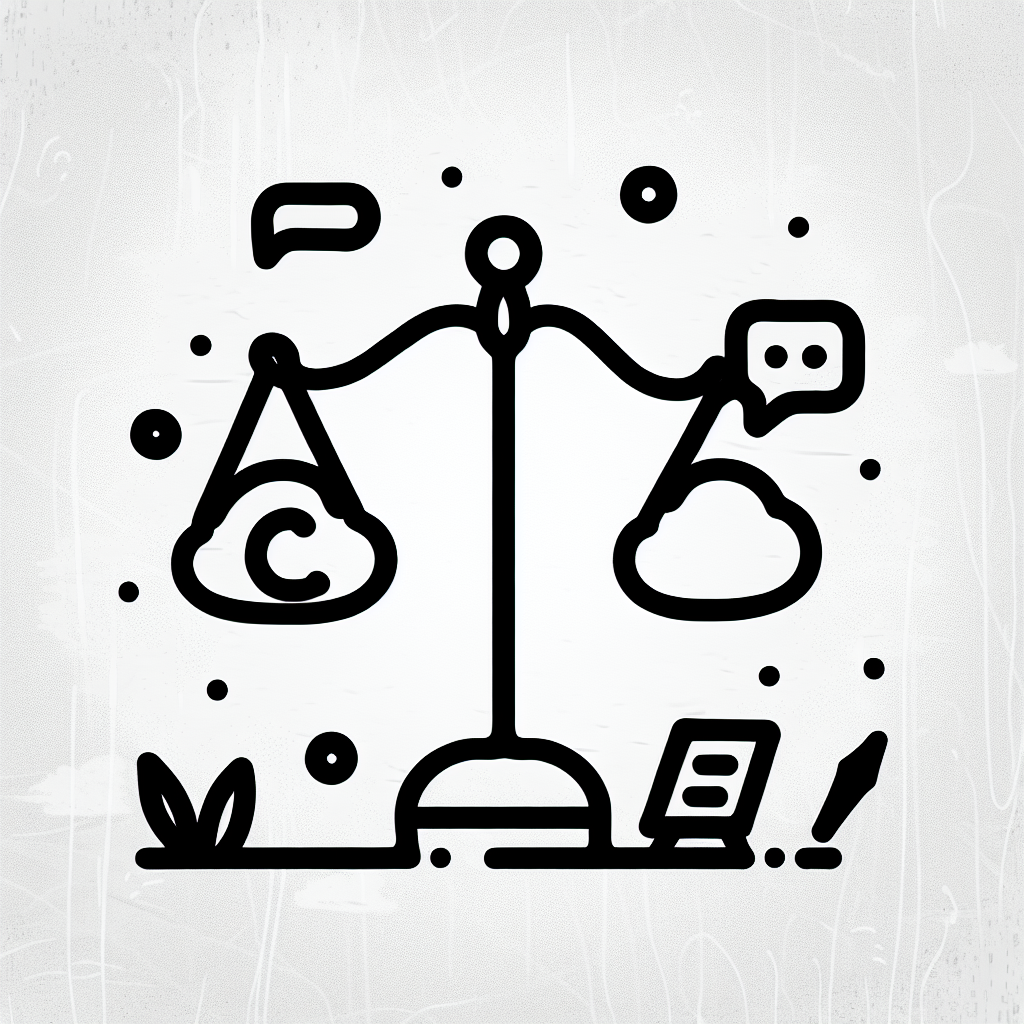
Criticisms and Challenges Facing YouTube's Algorithm
Transparency and Accountability
A significant criticism of YouTube's algorithm is its lack of transparency in decision-making processes. Users, creators, and researchers often struggle to understand precisely how content recommendations are generated. The opacity of these systems makes it difficult to evaluate the fairness or neutrality of recommendations, fueling concerns about potential biases and unintended consequences.
Due to this lack of transparency, there have been growing calls for increased accountability from YouTube. Advocates emphasize the need for clearer communication about the criteria used for recommending content and for stronger mechanisms to audit and explain algorithmic decisions. Enhanced accountability would not only build trust with users but also help mitigate unintended harms stemming from algorithmic recommendations.
Manipulation and Ethical Responsibilities
Another critical issue facing YouTube's algorithm is its susceptibility to exploitation and manipulation. Creators and actors with malicious intent can employ strategic methods—such as clickbait titles, misleading thumbnails, or coordinated engagement—to artificially boost visibility and influence the recommendation system. This manipulation can distort user perceptions, amplify misinformation, and negatively impact the quality of content available on the platform.
YouTube bears significant ethical responsibilities regarding the content amplified by its algorithm. Given the platform's enormous influence and reach, the company must proactively consider the ethical implications of its recommendation system. Developing robust guidelines and safeguards against algorithmic exploitation is crucial. By embracing ethical responsibilities, YouTube can better ensure that its algorithm promotes informative, reliable, and socially responsible content.
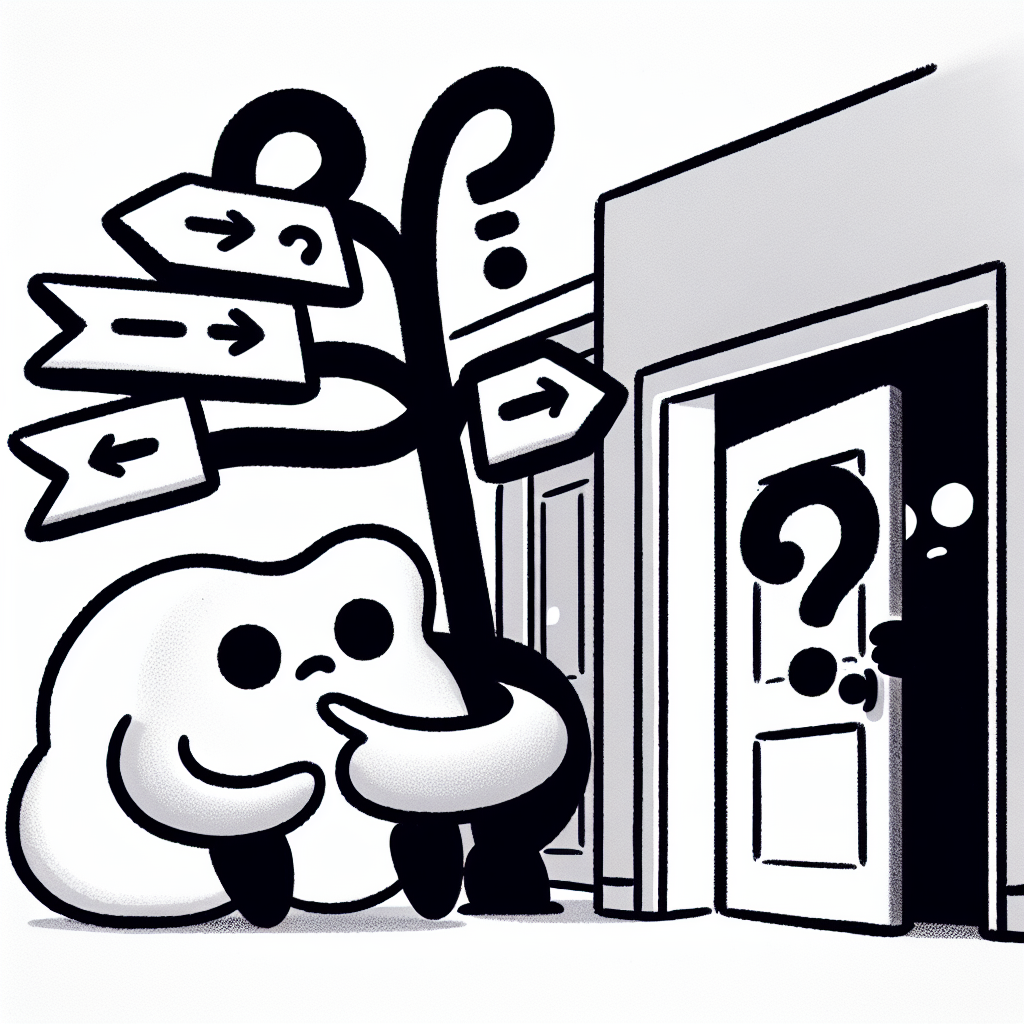
Future Directions for YouTube's Algorithm
Improving Transparency and Fairness
In response to ongoing public and regulatory scrutiny, YouTube is increasingly focusing on initiatives aimed at greater algorithm transparency. Efforts include clearer explanations of how content recommendations work, sharing insights into algorithmic decision-making processes, and providing users with more control over what content appears in their feeds. Such steps help users better understand why certain videos are recommended, which can build trust between the platform and its audience.
Balancing user engagement with responsible content promotion remains a crucial consideration. YouTube faces the challenge of keeping users engaged without inadvertently promoting harmful or misleading content. Future algorithm updates will likely emphasize responsible content moderation, prioritizing accuracy, reliability, and safety while still maintaining viewer interest and platform growth.
Technological Innovations and Future Trends
AI advancements will significantly shape the future trajectory of YouTube's algorithm. Enhanced machine learning technologies, such as deep neural networks and natural language processing (NLP), promise more precise content categorization, improved personalization, and more effective identification of inappropriate or misleading content. As AI becomes increasingly sophisticated, algorithms could adapt in real time to evolving user preferences and behavioral patterns.
Looking ahead, future algorithm developments may involve deeper integration of context-aware technology, enabling recommendations centered around the user's specific situation, location, or even emotional state. Additionally, predictive modeling could evolve to anticipate content trends and user interests more accurately, proactively suggesting videos that align closely with emerging preferences. Overall, these technological innovations indicate a future where YouTube's algorithm is more responsive, nuanced, and aligned with both user satisfaction and responsible content standards.
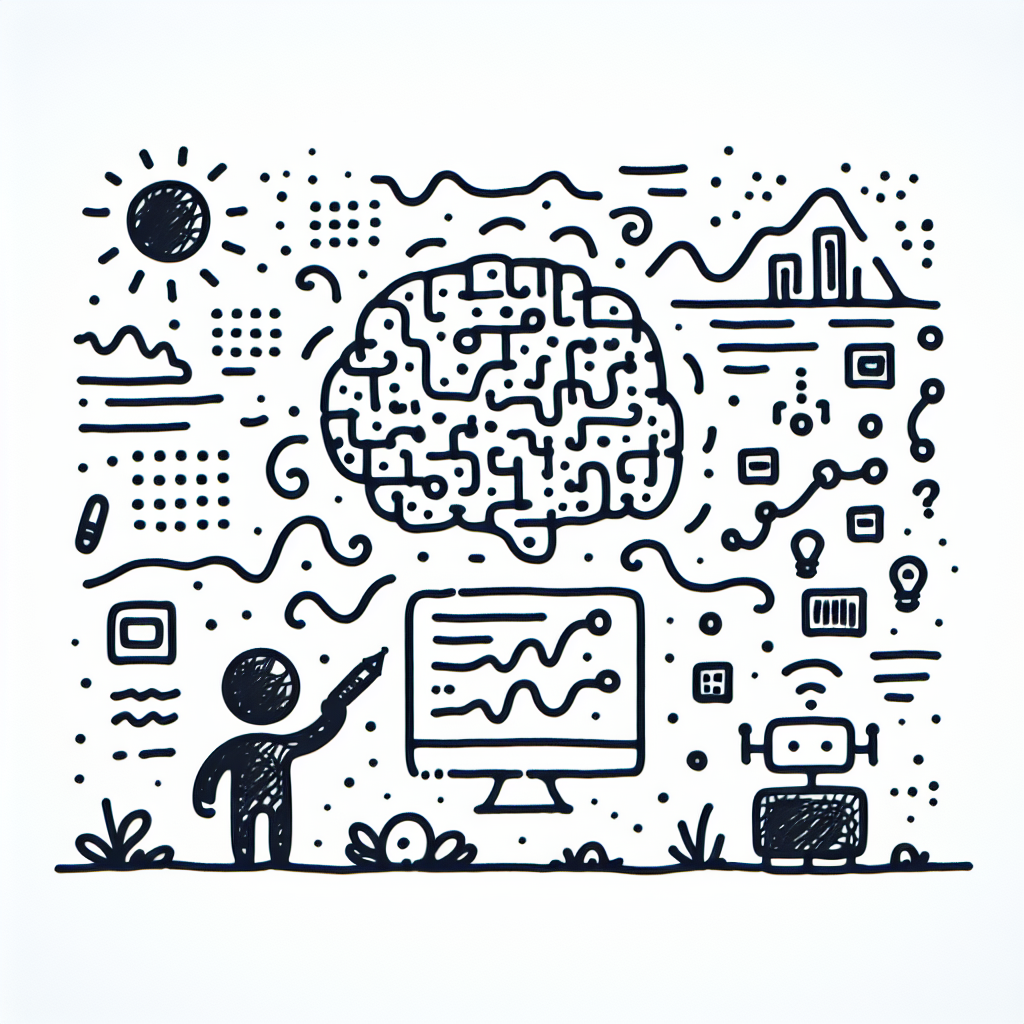
Conclusion
Key Takeaways for Viewers, Creators, and Platforms
Understanding the dynamics of YouTube algorithms benefits all stakeholders—viewers, creators, and the platform itself. For viewers, awareness of how algorithms influence content recommendations can lead to more intentional and satisfying media consumption. Creators who grasp algorithmic principles are better positioned to create engaging content, optimize visibility, and connect meaningfully with their audience. For platforms, transparent communication about algorithmic practices can enhance user satisfaction, engagement, and trust.
Navigating algorithmic challenges involves recognizing changing patterns, adapting strategies, and remaining informed about platform updates. Leveraging these insights creates opportunities for sustainable growth and improved user experience.
Final Thoughts on Balancing Algorithmic Efficiency and Ethical Responsibility
While algorithmic efficiency is essential for personalized and streamlined content experiences, ethical responsibility is equally critical. Platforms must prioritize user well-being and fairness, ensuring their recommendation systems avoid unintended biases, misinformation amplification, or harmful content promotion. Transparency and accountability in algorithm design and implementation are fundamental to maintaining user trust and fostering a thriving digital community. Balancing efficiency with ethical responsibility ultimately supports long-term, sustainable growth for YouTube and the broader digital ecosystem.
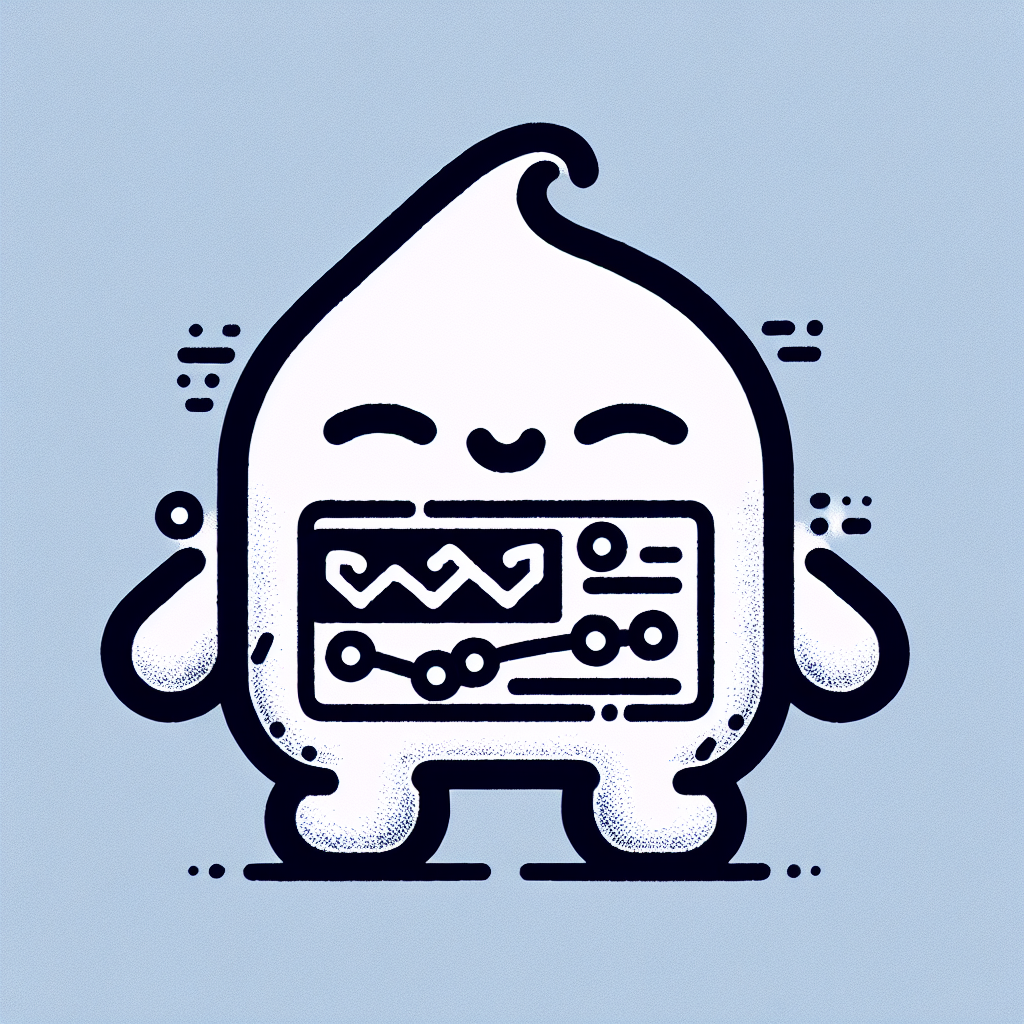
Land Your First Data Science Job
A proven roadmap to prepare for $75K+ entry-level data roles. Perfect for Data Scientist ready to level up their career.
Related Articles
Continue your learning journey with these related topics
Master Data Science in Days, Not Months 🚀
Skip the theoretical rabbit holes. Get practical data science skills delivered in bite-sized lessons – Approach used by real data scientist. Not bookworms. 📚